SEO click through rate research
MANY of us have seen SEO click through rate (CTR) studies, performed on large data sets, but what can we learn from these, and, more to the point, are they truly representative? Given the ever changing nature of the SERPs – are click-through rate (CTR) studies too crude and limited in their scope to cater for the multi-faceted nature of a typical SERP? And in fact is there even such a thing as a typical SERP anymore?
Taking the above questions and the previous studies as a starting point and also thinking about the factors influencing the human user, their query and their intentions; in this post I explore a little about what it all means.
What we have seen so far
Several studies have already been performed looking at click through rate (CTR) over the last few years on this topic. So, how did they go about it?

Optify assumed that all searches resulted in a top 20 click while the Slingshot SEO study calculated CTR using the number of Google Analytics visits divided by AdWords search volumes.
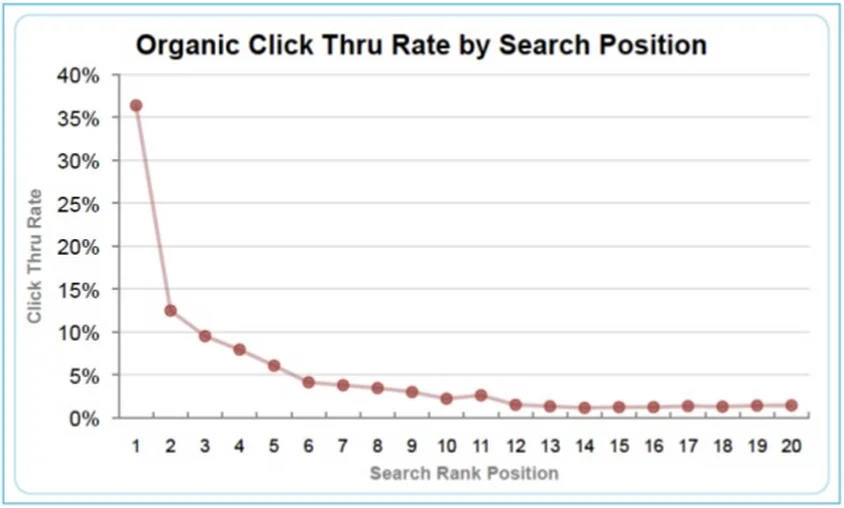
Meanwhile, Catalyst and AWR defined CTR as the percentage of impressions that resulted in a click using data from Google Webmaster Tools. In these studies they plotted the mean CTR against, “exact”, “average” or “search rank” position.

How will CTR help me?
It is relatively straightforward to generate an online market share model using Analytics SEO’s keyword tracking tool. A simple CTR model can be applied to search volume data and rankings for each of your and your competitors’ keywords to forecast clicks (volume of visits). This is extremely powerful.
What we did
From our client database, we took data for the 1,153 websites who have GWT data accounts connected to our platform. Search rankings were all “organic”, not universal and analyses independently of territory. We then proceeded to segment the data by various sub-categories, some of which the other studies had not recognised.
The chart below shows a sample of our raw data. When we produced our own mean curve, it looks unsurprisingly, very, very similar to the others.

In all, we found the underlying data to be quite widely distributed about the mean (shown in blue); taking a closer look at the distribution shows a wide spread. The two grey lines in the graph below indicate the range of 50% of the data points. The median line is plotted in black; this is the middle of the data set.
What does this mean? That there is huge variation in the data and a mean line alone isn’t representative.
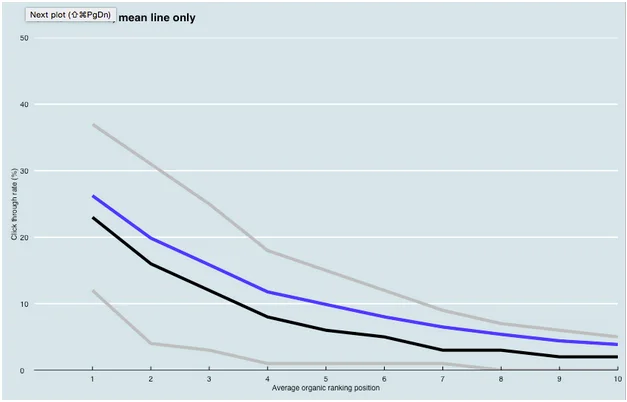
Segmentation
One common theme that we noticed in the other studies was the attempt to “segment” the dataset by certain factors. In order to better understand what really makes the user ‘click through’ the following factors were incorporated; whether the search intent was for a brand or not, the number of words per keyword phrase, and the source device type being a mobile or desktop.
We did the same segmentations and saw similar results- unsurprisingly. So, what next? Whilst we acknowledge that we can’t measure two key influential variables; the user’s state of mind and Google’s algorithm itself, we were able to add a few more metrics that we thought were easily obtainable and also missing from the previous studies.
The large website effect
The first interesting finding we discovered was that impact of the size of the pool of ranking keywords for a particular domain affects click-through rate immensely, for non-brand searches in particular. The click through pattern is so remarkably different that it should not be ignored.

Below you can see the large website effect for non-brand terms.
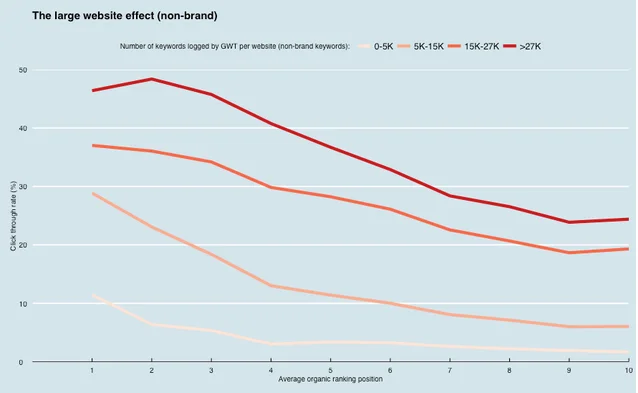
The long tail effect
The second non-standard finding that we can report is that variation in the number of impressions served (i.e. the number of searches logged) for a keyword has a significant impact. Greater numbers of impressions per keyword seem to reduce the magnitude of CTR across all positions in the top 10.

This phenomenon was not just true for our clients’ non-brand GWT data, AWR’s publicly available data set exhibits near identical properties. AWR chose a cut-off for their study; and only considered data points where the number of impressions was greater than 50. Here you can see the impact of changing that cut-off level.

This variation, seen clearly in both datasets may be related to the nature of the keyword phrases searched for. So-called “long tail” keywords, the rarer kind, attract far lower search volumes but may, conversely, attract a higher proportion of click through events than more commonplace keywords would. One reason for this increased CTR might be reduced competition in the same space (i.e. less choice).
When very high search volumes (greater than 1 million impressions served) are observed then we see click through behaviour similar to that for brand keywords; steep slope and much higher CTR at position 1.
Avoidance of weighting the data
In addition to the above, we noticed that our dataset was by no means evenly distributed between all sub-categories. We suspect that the other studies also suffered from this phenomenon. The sample size in terms of “impressions served” for our data set implies that our “mean” CTR values will be skewed by the biggest underlying class. An famous example of the resulting impact of not weighting data by sample size is well documented here.
As we have a majority of desktop, non-brand, single word searches in our sample- why should this be allowed to skew the reported CTR? On the other hand, perhaps our “sample” of client data is an accurate snapshot of universal clicking behaviour, and we should reflect these proportions in the data presented.
Finally, if the number of impressions served actually has an impact on CTR itself (i.e. the user sees “Amazon” more frequently in results, starts to trust the site more); we should not use it as a weighting factor, rather a segmentation factor. In fact our conclusion was that any CTR data should be heavily stratified; broken up into as many dimensions as possible.
Here is a sample of our segmented CTR findings in the table below. All values are percentages.